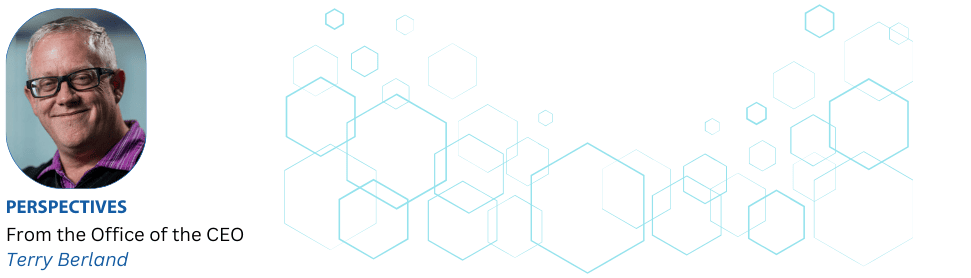
Maximizing Business Potential: Focusing on Outcomes-Based vs. Capabilities-Based AI Strategies
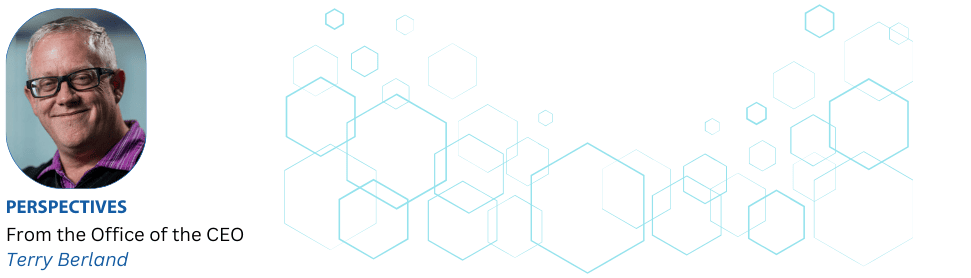
Artificial intelligence (AI) is revolutionizing the way businesses operate, enabling them to solve increasingly complex problems. However, companies are exploring the opportunities for using AI in two distinct ways:
1) Start with clear business problems you want to solve and then find AI solutions to help you—known as an outcomes-based AI strategy—or
2) Start with the exploration and acquisition of AI tools and then figure out how to deploy them—known as a capabilities-based AI strategy.
Understanding the distinctions between these approaches is crucial for any business aiming to leverage AI effectively. At LoadSpring, we have a strong view that one approach is far superior to the other.
Outcomes-based AI focuses on achieving specific, predefined results through the application of AI tools and techniques. Businesses adopting this approach typically start with a clear objective, such as increasing sales by 20% or reducing operational costs by 15%. AI applications are then designed and trained to deliver these specific outcomes. For example, an AEC company may use outcomes-based AI exploration to optimize its supply chain, directly leading to improved project efficiency and cost savings, demonstrating concrete results in the near term. This approach is highly effective for organizations with well-defined goals, as it allows for precise measurement of AI’s impact and ensures alignment with the strategic objectives of the business.
On the other hand, capabilities-based AI strategies emphasize developing and integrating AI applications that enhance overall business capabilities, irrespective of a specific outcome. This approach focuses on building robust AI tools that can adapt to various tasks and challenges, providing a broad spectrum of benefits, many of which are still to be determined. For instance, an AI-driven chatbot placed on top of your project-based data can handle a wide range of natural language inquiries, improving project reporting and operational efficiency over time, and enhancing a business’s overall agility and adaptability, even without a specific initial performance metric. The problem with this approach, however, is the potential to spend a lot of money and time before realizing you’ve chosen the wrong path and need to reverse course. This is the risk experienced with many large-scale ERP systems that promised massive business improvements but failed to materialize because the company never concretely visualized where the benefits were going to come from, making it hard to hold anyone accountable when those results fail to appear.
While both approaches have merit, we strongly advocate that our customers focus on an outcomes-based approach to exploring AI. This approach forces businesses to establish clear, quantifiable goals upfront, ensuring a direct correlation between AI initiatives and business success. This makes the return on investment measurable in real-time, not just after a significant investment has been made.
At LoadSpring, we empower capital- and project-intensive organizations to understand and leverage these approaches so they can harness the full potential of AI to solve their most pressing business problems.