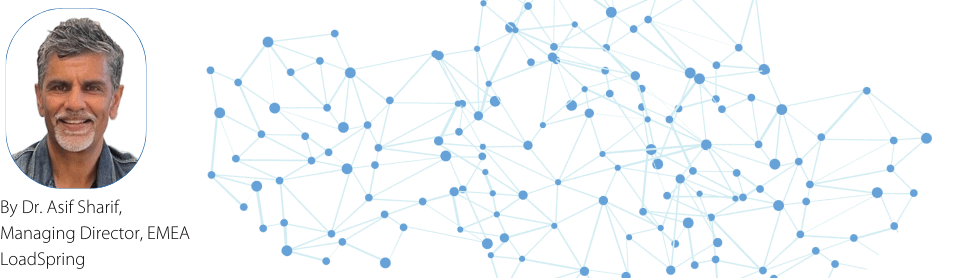
Bad Data, Bad Decisions: Why Data Hygiene is Crucial for AI/ML Success
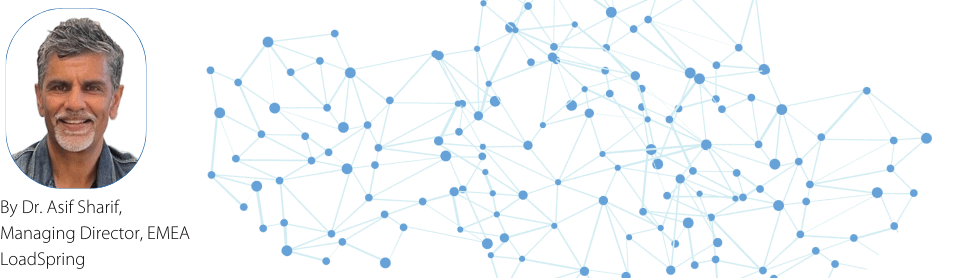
Artificial intelligence (AI) and machine learning (ML) technologies are rapidly transforming capital and project-intensive industries. These cutting-edge tools are not just optimising processes; they’re reshaping the entire landscape of architecture, engineering, and construction (AEC). Imagine streamlining complex design processes, enhancing project controls, planning, and scheduling, and automating construction tasks—all thanks to AI and ML. The possibilities for efficiency gains, cost savings, and innovation within the AEC sector are enormous.
Recent surveys reveal that more than 50% of AEC firms are already leveraging AI and ML in various capacities. What’s more, adoption is set to soar in the coming years as these technologies mature and become more accessible to all players in the industry. By quickly analysing vast datasets, uncovering patterns and insights, and offering data-driven recommendations, AI and ML are empowering construction and engineering professionals to boost productivity, minimise errors, improve safety and make more informed strategic decisions.
From generative design software that creates optimised architectural models in seconds to ML-driven predictive analytics foreseeing potential project risks and delays, the practical applications of AI and ML are as diverse as they are transformative. However, as reliance on data-intensive decision-making grows, it’s crucial to emphasise the importance of properly cleansing, governing, and preparing data assets. Only with high-quality data as a foundation can AI and ML truly deliver on their promise as game-changers for project-intensive environments.
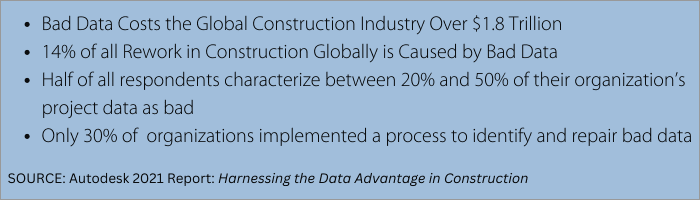
In essence, AI and ML are not just technologies; they’re catalysts for innovation and transformation in the AEC industry. As we move forward, embracing these tools and ensuring our data readiness will be key to staying ahead in an ever-evolving landscape.
AI and ML Rely on High-Quality Data
In the realm of AI and ML, data quality reigns supreme. These algorithms rely heavily on vast datasets to discern patterns, glean insights, and deliver accurate forecasts. The efficacy of AI and ML models hinges on both the volume and caliber of the data used during training.
Unlike traditional software, machine learning algorithms learn by analyzing large datasets to uncover relationships and make inferences. The richer the pool of high-quality training data, the more adept an ML model becomes at capturing intricacies and navigating complexities within the data. With large dataset, models continuously refine their accuracy, adeptly handling nuanced scenarios and offering dependable outcomes across varied real-world inputs.
However, the quality of data is just as important as the quantity. Training data must be clean, consistent, and complete for algorithms to learn effectively. Dirty data containing errors, duplicates, inconsistencies, missing values, or bias can significantly degrade model performance. Data cleansing and preparation are crucial steps before training AI and ML models to ensure the algorithms have a solid foundation to learn from.
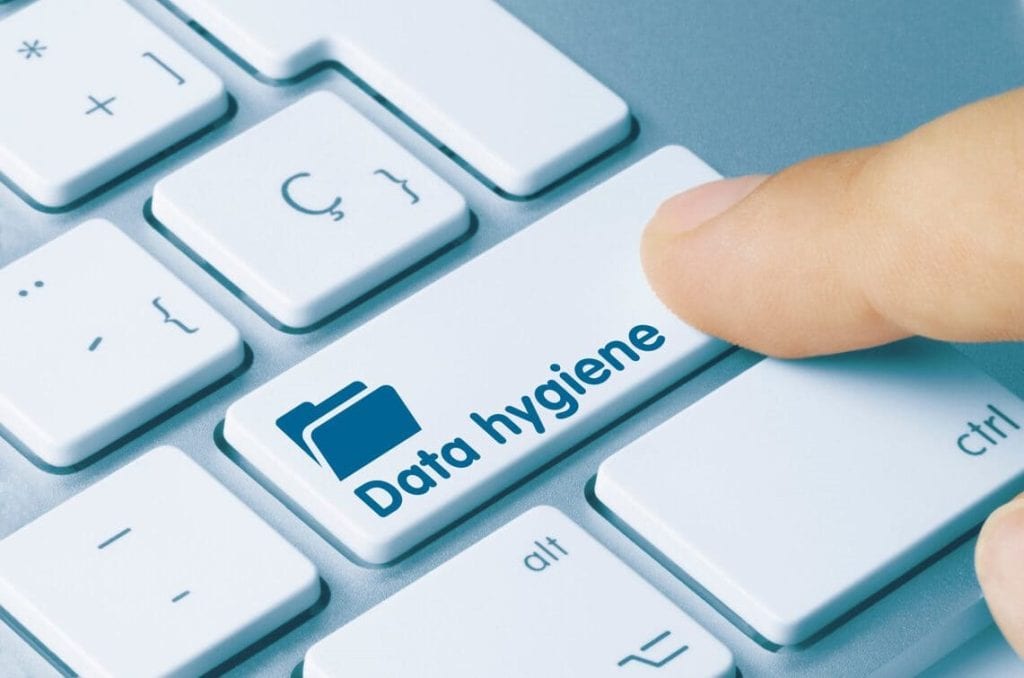
Real-World Data Is Often Messy and Incomplete
In the real world, raw data collected from various sources contains errors, outliers, and missing values that need to be addressed. When combining data from multiple systems through ETL (extract, transform, load) workflows, inconsistencies frequently arise due to differing formats, identifiers and human errors in data entry.
Data errors and anomalies occur for many reasons. Human error in data entry can introduce mistakes, equipment failures can cause sensor readings to be incorrect, and survey respondents may provide inaccurate or incomplete answers. Records can also be duplicated in multiple databases.
As data passes through various systems, variations in code, tags, abbreviations, and identifiers lead to misalignment. Date formats like MM/DD/YYYY versus DD/MM/YYYY, product codes with extra zeros added, or addresses entered differently are common sources of inconsistencies.
Problems also arise when information is missing entirely. Important attributes may not be tracked at all, fields may be left blank, and records may fail to be collected or get deleted.
All these data quality issues propagate downstream when multiple data sources are combined for analysis. The resulting dataset ends up with mismatched records, anomalies, gaps, and inaccuracies. Before data can be used for training AI and ML models, substantial cleansing is required to fix errors and fill in missing values.
Data Cleansing Is Essential Before Model Development
In data science, the adage “garbage in, garbage out” rings true. Flawed input data yields flawed outputs, highlighting the necessity of data cleansing before AI and ML model development. When it comes to developing AI and ML models, cleaning and preparing the training data is an absolutely critical first step.
Data cleansing enhances dataset quality by identifying and rectifying incomplete, inaccurate, or irrelevant data segments. This process ensures training data mirrors reality, enabling models to extract meaningful patterns.
Clean data fosters more accurate analytical outputs, crucial for valid AI and ML insights. Neglecting data cleansing compromises the integrity of AI and ML endeavors, emphasizing its indispensable role in preparing datasets for impactful model development.
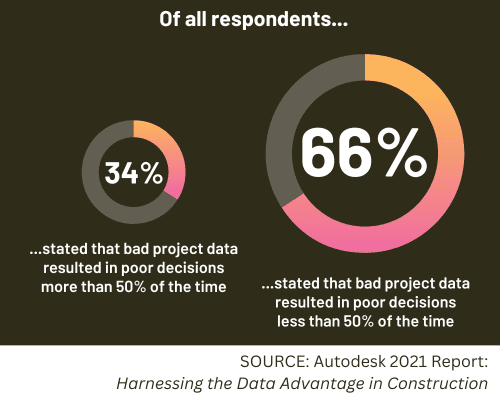
Consequences of Using Dirty Data
Utilising dirty, subpar data for AI and ML model training yields dire consequences, eroding trust and perpetuating flawed decision-making. Without robust data cleansing and governance, models inherit the shortcomings of the data.
Dirty data introduces inaccuracies, biases, and inconsistencies into model logic, yielding erroneous outputs and predictions. Systematic mistakes driven by dirty data exacerbate poor analysis and decisions, yielding rapid, poor-quality outputs.
Moreover, dirty data undermines trust in model outputs, eroding stakeholder confidence. When AI and ML models consistently err due to low-quality data, credibility suffers, leading to skepticism and reluctance to rely on model insights.
Conclusion
In summary, data is the lifeblood of AI and machine learning systems. Without adequate data cleansing and governance, these advanced technologies will fail to live up to their promise.
As raw data is often messy, inconsistent, incomplete, and full of errors, data cleansing through techniques like handling missing values, smoothing noise, correcting inconsistencies, and removing duplicates is essential.
If AI and ML models are built on top of dirty, low-quality data, the result will be inaccurate outputs, perpetuating and amplifying any existing data issues. This can lead to biased and incorrect decision-making.
Proper data analysis and cleansing technology, such as LoadSpring’s INSIGHT solution, are critical to the success of your AI/ML initiatives because they can transform your data into clean, accurate, reliable, relevant, and analyzable data that will inform your most important business decisions for less than the annual cost of an internal data analyst.
High-quality datasets are the fuel that powers AI and ML. By investing in robust data cleansing technology, organizations can harness the full potential of these transformative technologies to drive fact-based, ethical business decisions.
AI and ML success is only possible through diligent data preparation and stewardship.