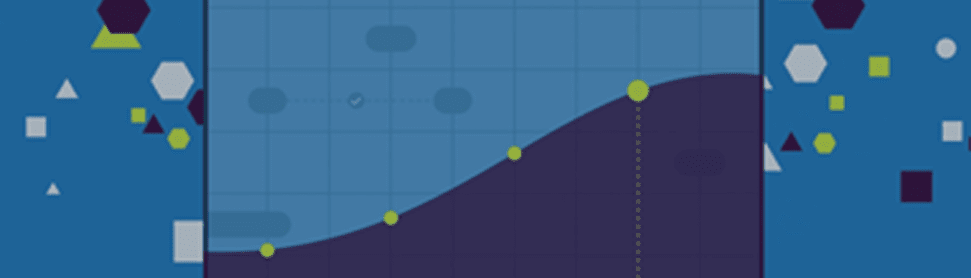
What are S-Curves in Projects & How to Automate Them to Save Time and Money?
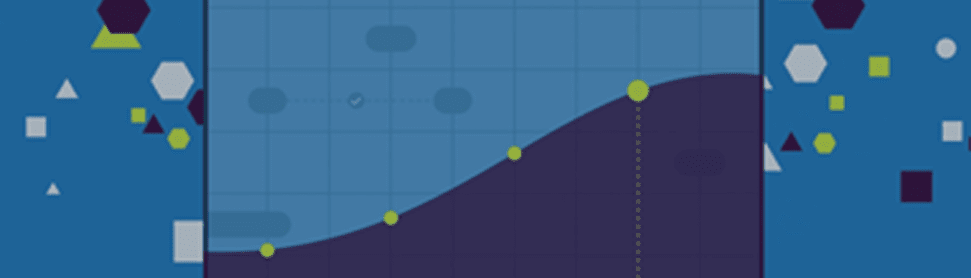
This article will define S-curves, explaining how automating them can save you time and money. We examine the connection between S-curves, project controls, and project management: exploring how to decide which questions to ask; factors that determine measurable metrics; and the advantage of S-curve automation. We’ll also demonstrate how easy S-curve automation can be with LoadSpringANALYTICS: Automated S-Curves.
What Are S-Curves?
An S-curve in project management refers to a mathematical graph representing all of the data for a project. The graph usually resembles an “S” because at the beginning of a project, during planning, progress is slow and steady, and eventually reaches an inflection point of increased project activity. Likewise, towards the end of a project, another inflection point is reached where the project progress slows while it winds down.
Creating S-Curves Takes Time & Money
If your company is creating S-curves today, you are spending many hours and a significant amount of money to make them. S-curves are essential for KPIs. Most companies read and rely on S-curves during a project, but they often become frustrated when there are scheduling or cost-related overages by project’s end.
Instead of taking time and energy away from onsite IT team members and data scientists, why not invest in a cloud-based platform that automates your S-curves?
LoadSpringANALYTICS: Automated S-Curves
LoadSpring’s proprietary data extraction process from your project control applications automates the creation and automation of your S-curve. Save your team time and endless frustrations by delivering and monitoring your S-curve in almost real-time.
Dashboards and visualizations of your team’s progress are essential to successful project controls. If you’re interested in seeing how this works for your company’s business objectives, LoadSpringANALYTICS: Automated S-Curves can automate your S-curves right now! We can quickly help your team set you up for success to clean and refine your data, allowing you to create a usable data lake with LoadSpring ProjectINTEL.
Why Should You Use S-Curves?
In plain terms, an S-curve is a logistical curve or mathematical model describing one variable’s growth in relation to another variable over time: such as budget variance—comparing projected costs with actual costs and the difference between the two—as well as how that budget variance changes, over time.
Or, as McKinsey & Company puts it, the S-curve framework is “used in various disciplines to represent the beginning, rapid growth, and maturity of something via an S-shaped curve.” Notably, they classify S-curves as an integral part of learning and development (L&D) for individual learners in their given roles.
This apparent disparity between individuals and metrics dissipates quickly upon examination: without the tools, resources, or motivation to carry out tasks and goals, metrics exist in a vacuum. The ongoing corporate juggling of so many projects prompted Harvard Business Review writer Antonio Nieto-Rodriguez to propose a new executive role: Chief Project Officer.
“Thirty years ago,” Nieto-Rodriguez writes, “80% of the resources in an organization were dedicated to operation, and 20% to projects; today, that ratio has flipped.”
Cloud project controls have become central to successful digital transformation and efficacy in achieving long-term forecasted goals. Why? Because the successful planning, execution, and delivery of projects is critical to business growth.
Why Are S-Curves Critical for Growth?
Because they allow you to visualize how your company is performing regarding chosen key performance indicators (KPIs), you can understand which KPIs may be slowing you down or need adjusting, depending on the resources allocated to a particular project. S-curves can also shed light on the relative level of maturity that a particular metric has achieved, allowing you to adjust measurement factors depending on the project’s needs as it progresses.
Harvard Business Review’s Graham Kenny claims that project KPIs should be based on the priorities of strategic stakeholders and that traditional KPIs fail to show concern for all interested parties. However, projects stand a much greater chance of succeeding if all stakeholders’ needs and goals are addressed and if all involved parties trust its objectives in the first place.
Data Distrust & Delayed Analytics
Here are the top three reasons why 77% of leaders don’t trust data:
- Data quality & formatting
- Siloed data sources
- Delayed analytics
S-curves often fall into the delayed analytics bucket of distrust because they are time-intensive to create regardless of the number of macros written and data team members involved. This is where automation comes into play as being helpful to achieving your S-curve goals.
Let us know what applications you are using, and we can automate your S-curves today.